Across professional services, companies are considering how they can apply AI — and many have already moved ahead.
Before a company employs its next AI solution, it should examine its proposed use cases against its business model, clients, data and risks. Today, most AI use cases in professional services can be found in three broad types of opportunities.
See the opportunities
Services firms have found many ways to drive business value with AI — consider where you might have these opportunities and needs:
- Efficiency and analysis
- Personalization
- Idea generation
Efficiency and analysis
Professional services companies sell time and expertise. So, if they make their time more efficient, they can reap financial benefits almost immediately. “Efficiency improvements typically involve the acceleration of manual and/or repetitive tasks,” said Grant Thornton Risk Advisory Services Principal Johnny Lee. “Opportunities for efficiency are what first attract most companies to a proof of concept for AI — and, in the services industry, those opportunities abound.”
Processes like time tracking and billing often involve repetitive manual tasks that consume significant back-office resources — and they can also pull time away from billable hours for your people who are client-facing.
Companies with on-site technicians can use AI technology to help collect and aggregate service data. “That's a lot of distributed data collection, which can be very repetitive work,” Lee said. For legal firms, architects and other office services, AI technology can drive efficiency in specialized analysis and related work:
- Research relevant legal cases, statutes and precedents.
- Apply eDiscovery across emails and other electronic information, intelligently summarizing the information or identifying items of interest.
- Review contracts and other documents in development or after receipt from outside parties.
- Analyze architectural designs, evaluating parameters and constraints to suggest solutions.
- Respond to inbound RFPs or aggregate responses to outbound RFPs.
- Model structures to help identify potential issues or opportunities for energy efficiencies.
- Manage projects dynamically, adapting complex timelines, dependencies, resources, budgets and other factors to reflect new changes or model multiple scenarios as you consider planning options.
“AI is good at helping you understand the data you already have,” Lee said. That can give you a business advantage in analyzing data from clients — but it can also help you improve services in other ways.
Personalization
Services firms can use AI technology to personalize experiences, content and communications for particular audiences. Consider use cases where personalization can help tailor your marketing, advertising, support or services:
- Analyze your current client base to refine customer segmentation that identifies the types of clients you have (and don’t have), their patterns, preferences and other details that can inform your client interactions.
- Anticipate client needs with proactive communications or services that you can offer to clients as those needs arise.
- Communicate with clients across targeted channels, with marketing campaigns tailored to reach them where they are — possibly even offering chatbots to directly answer questions or perform administrative tasks for routine requests at any time.
“I think the ability to identify the entire population of your existing customer base or potential customer base, and then segment that base, considering interests and patterns of behavior, ultimately targeting people based on those segmentations — that kind of personalization is a very attractive promise of AI,” Lee said.
“An analytical tool with AI technology can tell you things about your customer base that you didn't know, like two-thirds of your customers came from the same six ZIP codes, or two-thirds of your customers are in this age range. That may not be an analysis you’ve considered,” Lee said. “It’s also good at identifying new populations to consider, from broader demographic data.” Consider how you could tailor your promotional plan to yield the highest ROI on your investment. “These factors are all analyzed in traditional marketing efforts, but often with unreliable benchmarks and sampling methodologies that are mathematically questionable,” Lee said. “AI allows for more rigor. It allows for pattern analysis on a scale that's much more mathematically probabilistic and reliable than sample-based methodologies and traditional marketing, branding and advertising gambits.”
As you analyze more information about your business and its customers, you can move toward a new level of AI-driven insight — idea generation.
Idea generation
“Idea generation, which many strategists call ‘ideation,’ involves creating new and unique outputs based on useful hypotheses,” Lee said. This activity often involves building scenarios based on data from your company and your larger market:
- Measure and optimize business functions with technology that can analyze data to suggest improvements across your company’s marketing, HR and other functions.
- Analyze broader market conditions, to model the potential impact and revenue of territory expansion, M&A with other companies, or offering new services that are identified as unmet client needs.
- Examine your company’s business and financial data to highlight trends, predict outcomes and model scenarios that show potential returns on new services.
- Model, develop, test and maintain subscription-based services or other alternative delivery models.
Key insights along these lines typically unfold from broad-based questions, like “What other services are adjacent to our current offerings, and how might we consider bundling these — or perhaps even acquiring a competitor to bundle?”
“Idea generation is a very powerful promise of AI,” Lee said. In particular, GenAI offers a dynamic and responsive platform for exploring ideas — if you understand how to use it well. “In GenAI, it all comes down to the quality of the language model and the quality of the prompts for that language model.”
Understand the risks
Each AI use case category has unique risks. To manage these risks, you need a structured approach. There are many AI risk management frameworks, and you can choose one based on your regulatory, business model and cultural requirements.
“There are already more than 40 published frameworks to address risk management for AI,” Lee said. This number is partly due to the variation in the regulatory requirements that govern AI use around the world. Globally, hundreds of regulations have been published, adopted and codified. While the US has not enacted federal laws related to AI technology, as of August 2024 there are 21 states with some form of enacted AI legislation and 14 additional states with proposed legislation in committee or beyond.
Most of the AI risk management frameworks address similar categories of risks. “There are about a dozen risk categories that come up again and again,” Lee said. The most common risks can be categorized into three types:
- Design
- Consistency
- Trust
Design
“The technical design characteristics are the things under the control of AI system engineers and developers. In that category, one of the most consistent risk domains is accuracy — you'll see that when you read about AI failures,” Lee said, citing anecdotes like the attorney who submitted a legal brief to the court citing fictional case law.
Other consistent risk domains include considerations for cybersecurity and data privacy. For any AI solution — especially those accessing your data — you must verify that cybersecurity and data privacy risks are managed in a way that can satisfy your regulatory and reporting requirements. Put differently, it’s crucial to form a strategy that specifically addresses the cybersecurity risks in AI.
Consistency
Businesses need reliable results. “What is the consistency of the output?” Lee asked. It’s easy to understand the appeal of a GenAI solution that dynamically generates answers from everything it can find. However, that also means the answers will change when the solution finds new information — for good or bad.
“All GenAI models experience the concept of ‘drift,’” Lee said. GenAI outputs will change, based upon the quality of the language model and the prompting employed to interrogate that language model. “The concept of drift refers to these changes in output, which can be both subtle and occult. Over time, drift can produce inconsistent results — meaning you interrogate it with a specific prompt on Monday, then you enter the same prompt on Friday, and you get a different answer. Absent human quality control, that inconsistency can be either welcome or deeply troubling.”
When an individual asks a question and GenAI has a different answer from last time, that’s interesting. For businesses, that’s not interesting. Businesses need to know that the same question will elicit the same answer, unless and until that answer needs to change.
In a business context, reliability factors into resilience, security, transparency and other requirements. In fact, some malicious attackers use the phenomenon of drift as a point of attack. “There's a concept called ‘model poisoning,’ where malicious actors deliberately try to mis-train the language model, feeding it garbage prompts and training it to produce inconstant or deliberately incorrect outputs,” Lee said. These risks can result in dangerous outputs and ultimately erode the trust in an AI solution.
Trust
“The third category of high-priority risk characteristics are what I would consider lodestar concepts of trustworthiness,” Lee said. “Is this fair? Are we reinforcing things that are inequitable in an existing system? Think of something like predatory lending. Are you removing accountability from the responsible parties, including biases baked into the model but hidden from the user?”
To ensure the fairness, transparency and accountability that trust requires, you must understand the data driving an AI model. “You have to understand on which data you're getting outputs,” Lee said, adding, “If the language model isn't tailored to meet your needs, then you're just using the wrong product. This is analogous to other technology adoption phenomena, of course, but if you’re not clear on the requirements, then you're inviting risks in a way that might prove dangerous. For AI adoption, you’ll need to ensure that you're not giving away IP rights, violating someone's privacy, or creating new liabilities for your organization.”
Manage your approach
AI technology, and the required risk management, can seem like a complex proposition for a small or midsized professional services firm. That’s why some firms look for managed third-party AI services where the risks and controls are already in place.
“It's common,” Lee said. “A lot of our clients are saying, ‘We don’t have the staff or infrastructure to handle this nuanced, complicated technology. So, can’t we just find a vendor who can help us do this?’"
“While I understand that inclination, it's a dangerous formulation,” Lee said. “The most dangerous part is the implicit part — the things that you're not considering before you start.” Companies need to ask a series of questions before they bring AI capabilities into their environment.
Isolation
“The first question to ask, perhaps above all the others, is: ‘Are we operating within a walled garden?’” Lee said. Consider the developer’s maxim that, “If you aren’t paying for the product, then you are the product” — most free services pay their expenses by monetizing the data and other information they collect from users.
“If you just go to a free GenAI interface and enter proprietary information, you’re giving that IP away in ways that are pretty occult, hard to track and impossible to recover,” Lee said. “You shouldn't blindly trust vendors, especially the less-established ones, to always share the truth about that.”
Stakeholders
While business areas must take ownership of their technology solutions, they must also consult other areas before employing a new AI solution. Every AI solution needs to be accounted for within the organization’s risk management and governance.
“Like cybersecurity, AI governance done well is a multidisciplinary approach,” Lee said. “Identify the stakeholders who could be impacted by this — like employees, customers, vendors, downstream providers and third-party IP holders.” Then, include HR, legal, finance, IT, operations, compliance and other teams, to identify the use cases, relevant metrics, risks and other issues.
“Harkening back to the can’t-we-just-hire-a-vendor for that commentary, you can't outsource that,” Lee said. “As you adopt the proof of concept, it's important that key stakeholder perspectives are contemplated for proper risk management. And you need to have an internal champion.”
Human oversight
Your organization needs input from across the range of stakeholders for an AI solution implementation — but it also needs human oversight for the solution’s outputs.
“If you look at all of the anecdotes of AI technology gone wrong, it's the absence of a human overseer every time,” Lee said. “You need to have a human overseer to make sure the solution is telling the truth, and confirm that it's providing utility as opposed to sending you down some expensive liability nightmare.”
Third-party AI services come with the same warning as third-party cybersecurity services: You can outsource the solution, but you can’t outsource the risks; those belong to your organization, so they must be contemplated and addressed by those within your organization.
The risks are still yours.
“I think that's the most essential insight,” Lee said. “You can have advisors help identify a proof of concept, but if you don't dedicate someone to oversee the outputs — to be accountable for the quality, consistency, and reliability of same, it can lead to a very bad turn of events. Not only can you not give the system itself a long leash, you should never let it completely off the leash.”
When you understand your AI opportunities and risks, then you can form the risk management and governance framework you need. This framework will help bridge the risks between today’s needs and tomorrow’s AI opportunities.
Contacts:
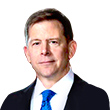
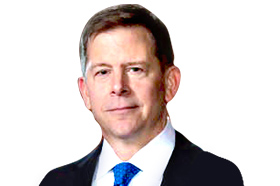
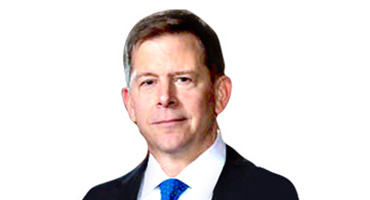
Frederick J. Kohm
National Managing Principal, Services Industry
Principal, Risk Advisory Services
Grant Thornton Advisors LLC
Frederick J. Kohm, Jr. has over 26 years of experience providing accounting and advisory services to his clients.
Philadelphia, Pennsylvania
Industries
- Insurance
- Energy
- Services
Service Experience
- Advisory
Content disclaimer
This Grant Thornton Advisors LLC content provides information and comments on current issues and developments. It is not a comprehensive analysis of the subject matter covered. It is not, and should not be construed as, accounting, legal, tax, or professional advice provided by Grant Thornton Advisors LLC. All relevant facts and circumstances, including the pertinent authoritative literature, need to be considered to arrive at conclusions that comply with matters addressed in this content.
Grant Thornton Advisors LLC and its subsidiary entities are not licensed CPA firms.
For additional information on topics covered in this content, contact a Grant Thornton Advisors LLC professional.
Our fresh thinking

No Results Found. Please search again using different keywords and/or filters.
Share with your network
Share