Generative AI drives value across portfolio companies
The potential for data science and advanced analytics, including AI, to generate both pre-and post-deal value for private equity (PE) investors is immense. Despite the vast research and literature on this topic, our experience proves that sophisticated PE firms have only started to scratch the surface in incorporating these capabilities into their value creation roadmaps and applying them across their portfolio companies.
Innovative PE firms start their analysis of the value case for advanced analytics as early as target screening. These firms are leading with a data-first playbook and prioritizing the review of their target’s data science and analytics capabilities during diligence. The most common findings, at least in the middle market space, are targets with immature to non-existent data organizations. These companies are often sitting on loads of data, but not utilizing it to improve or grow their business.
Low-hanging fruit
PE firms that have prioritized advanced analytics are often starting with very similar use cases. Some of the most common areas that can immediately move the needle include:
- Financial use cases
- Cash flow forecast — Using analytics to estimate the flow of cash in and out of the target over time to help predict future cash positions and to avoid crippling cash shortages.
- Accounts receivable/accounts payable — Analysis to keep track of receivables, streamline collections and provide insights into payments.
- Commercial use cases
- Pricing dashboard — Allows pricing and marketing teams to view and understand how pricing affects the business in terms of sales volume, revenue, gross margin, etc.
- Market size and white space — Analyzes an existing market to identify which products or services are succeeding, which are failing, and where potential gaps may exist, to uncover opportunities for growth.
- Customer profitability — Looks at the revenue (or profit) that each individual customer generates, incorporating customer acquisitions costs, frequency and value of custom purchase, costs of goods sold, etc.
- Product profitability — Identifies the profitability of the product portfolio at the product level to execute on product rationalization, opportunities for expansion, etc.
- Other use cases
- Cyber risks — Provides details about the number of data breaches, records exposed, industry type, breach type, threat vectors, data type, geo-location mapping, costs, etc., incorporating AI to enable more proactive addressing of cybersecurity threats.
- Deal team effectiveness — Uses analytics to assist with sourcing acquisition targets and measuring deal team productivity.
Despite the value creation opportunities for these and other use cases, there are a few factors that are holding companies back. The first is the availability of skilled people who are able to execute on advanced analytics projects to deliver benefits to the organization. If people with these skills don’t exist within the organization, it may be necessary to recruit from elsewhere to support advanced analytics initiatives and the improvements they can bring.
Companies sometimes work with third parties to get started on advanced analytics until they can build up their own capabilities internally. Ultimately, the goal usually is to have an internal chief data officer who has experience building out a data science function and the ability to recruit the capabilities to handle advanced analytics into the organization.
Second, some organizational leaders think they have to get all their data into one place before they can apply analytics. The job is so big that the projects never get off the ground.
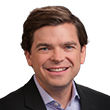
“Customer and product profitability are often the use cases that companies prioritize first on their advanced analytics agenda.”
Smaller and mid-sized organizations may be able to embark on simple yet impactful data analytics projects without taking such an overwhelming and comprehensive approach. It’s likely that helpful and significant data already resides within their ERP systems, which often come equipped with capabilities that enable the immediate generation of reports and analytics that can make a difference for their organization.
Sometimes these benefits already are available to companies that learn how to take advantage of the tools that already exist and develop the discipline and focus to perform the data science to determine what reports will benefit the business — and then develop those reports.
“Customer and product profitability are often the places where companies start with advanced analytics,” said Tim Ziemann, Senior Manager, Transactions for Grant Thornton. “The data is often already available to support these uses cases in a way that can bring immediate benefits.”
Management’s role
Your CEO is the most important ally in starting your data science and advanced analytics roadmap. As with any investment, you’ll need to quantify the business case for the initiatives on the roadmap and clearly link the analytics use cases with the business benefits.
As with any IT initiative, the best practice often is to link advanced analytics initiatives to important strategic goals that the organization is pursuing.
“If the company’s goal at the highest level is to grow revenue or grow profitability, you can link some of these use cases directly to that goal,” Ziemann said. “You can quantify the percentage of revenue or profitability increase that you expect if you adopt certain use cases using data analytics.”
Showing these links clearly can be a challenge, and some will argue that advanced analytics initiatives aren’t quantifiable, which just isn’t true. Given all the money being spent on analytics, there is surprisingly little scrutiny about their impact. The fundamental questions you must always inquire is the following: “Have I chosen the right business problem with real and meaningful (+ ROI) impact on the business?”
The next frontier
Data science and advanced analytics is a huge industry and is expected to keep growing at a steady pace. The industry will continue to disrupt the market and provide a competitive advantage to organizations that stay abreast and embrace the latest trends. One of the most prevalent trends cited by prominent PE investors is conversational analytics, or using AI and specifically natural language processing to analyze customer conversations and use them to understand their sentiment, intent and interest.
Other trends that we’ve discussed with our PE colleagues include chat interfaces that prompt the right questions, the ability to enrich current data sources from data retailers, and solutions that address the “last mile” problem — an issue where the data science and advanced analytics capabilities are available, but just aren’t being utilized by leadership or the broader organization. The advancement in conversational analytics has the potential to unlock access to the data gold mine for a much larger population of people within the enterprise, bringing exponential value to all functions.
The past year has seen a transition where generative AI models have become generally available in addition to the existing traditional AI tools.
The capability of large language models to interpret language and questions — and to generate content — has increased significantly in the last six months. The technology can be applied to a variety of formats, including text, images and voice.
The advancement in these tools creates numerous new use cases for many more people. It also creates new governance imperatives, as organizations need to fully understand both the benefits and the risks associated with this technology and its algorithms. Governance over these tools and an organization-wide plan is essential, with oversight from both management and the board.
Where to start
Getting started on incorporating analytics into current value creation roadmaps requires taking stock of the data, data sources and advanced analytics capabilities that currently exist in the organization. Whether an organization is investing in a data lake or associated infrastructure — or using its current applications — parallel activities should include procuring an analytics boutique firm to begin helping to define a few use cases (and the associated ROI) and hiring a data leader to begin charting the course.
As is the case with any capability, there’s an advanced analytics maturity curve, and it can be six months or more before enough muscle has been developed to see measurable results. But the opportunity is significant, and organizational leaders should be mindful that if they don’t take advantage of these capabilities, they might fall behind competitors who will.
Contacts:
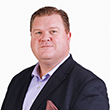
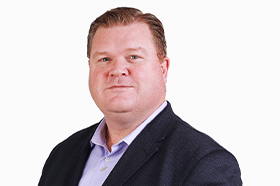
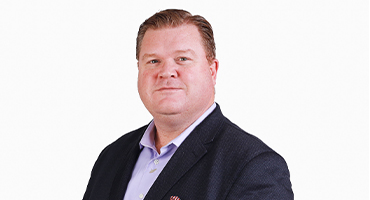
Richard J. Sittema
Principal – Transaction Advisory Services, IT Transaction Advisory, Healthcare and Manufacturing Practices
Grant Thornton Advisors LLC
Richard Sittema has more than 15 years of experience in business consulting. He started his career in business analyst roles on large-scale systems integration and IT-related consulting engagements for public, private and not-for-profit organizations.
Cleveland, Ohio
Industries
- Manufacturing, Transportation & Distribution
- Not-for-profit & higher education
- Healthcare
Service Experience
- Transaction advisory
- Technology modernization
- Risk advisory
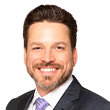
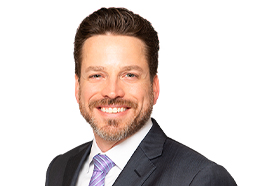
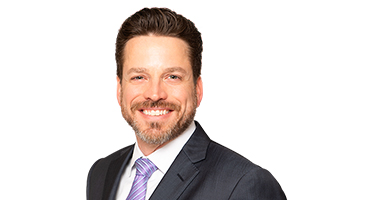
Will Whatton
Principal, Technology Modernization Services
Grant Thornton Advisors LLC
As a Principal within our Technology Modernization practice, Will leads our Enterprise Data capabilities focused on maximizing enterprise data value through data strategy, data governance, data engineering, data analytics and advanced data capabilities such as Artificial Intelligence and Data Science.
Houston, Texas
Industries
- Not-for-profit & higher education
- Healthcare
- Energy
- Life sciences
- Construction & real estate
- Manufacturing, Transportation & Distribution
- Asset management
Service Experience
- Technology modernization
Our private equity featured industry insights

No Results Found. Please search again using different keywords and/or filters.